Data Analysis Demystified: Simple Strategies for Better Results
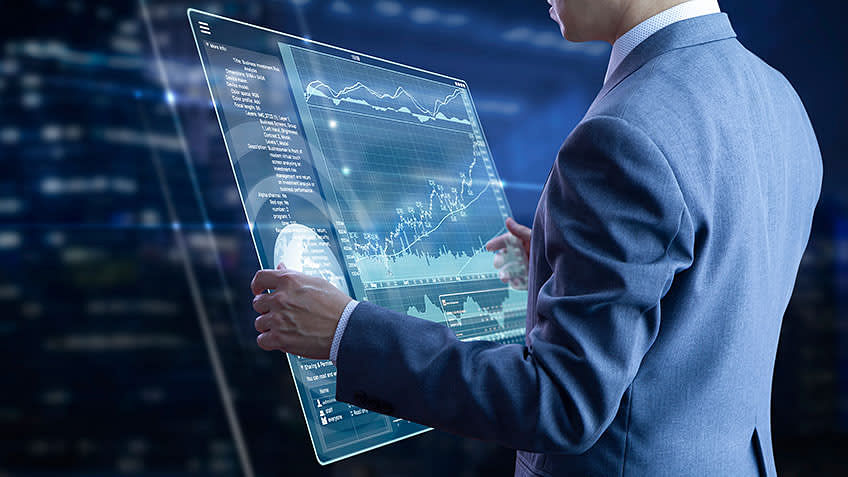
data analysis In the vast landscape of research and business intelligence, data analysis is the beacon that transforms raw information into actionable insights. Whether you’re sifting through customer feedback, scientific experiments, or market trends, effective data analysis is crucial for making informed decisions and achieving success. However, the process can often seem daunting, filled with complex jargon and intricate methodologies. This guide aims to demystify data analysis with simple strategies that can help you achieve better results and unlock the full potential of your data.
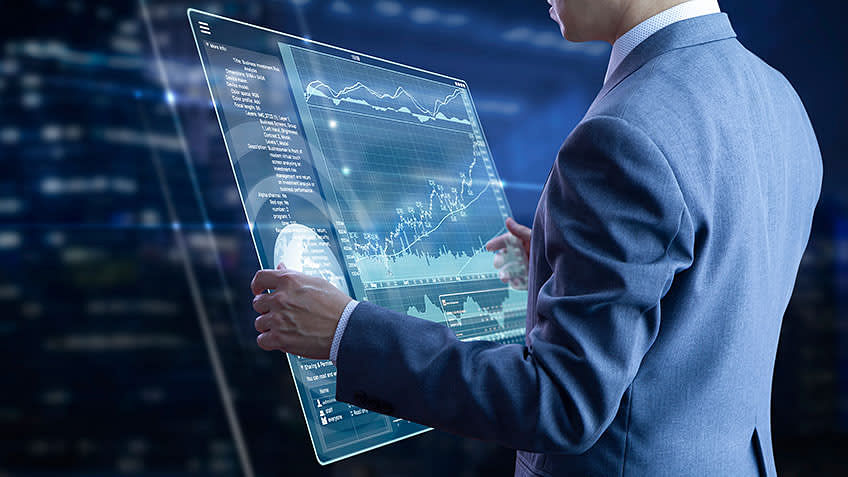
The Essence of Data Analysis
Data analysis is the process of systematically applying statistical and logical techniques to describe and evaluate data. Its primary goal is to extract useful information, draw conclusions, and support decision-making.
What is Data Analysis?
Data analysis involves several key steps:
- Data Collection: Gathering relevant data from various sources, such as surveys, experiments, or databases.
- Data Cleaning: Preparing the data for analysis by handling missing values, removing duplicates, and correcting errors.
- Data Exploration: Examining the data to understand its structure, patterns, and relationships.
- Data Interpretation: Applying statistical methods to interpret the data and draw meaningful conclusions.
These steps form the foundation of data analysis and ensure that the results are accurate and actionable.
Key Components of Effective Data Analysis
To achieve better results in data analysis, it is essential to understand and apply the core components of the process. Each component plays a critical role in ensuring that the analysis is thorough and insightful.
1. Defining Clear Objectives
The first step in data analysis is to define clear objectives. This involves specifying what you want to achieve with the analysis and what questions you need to answer.
- Identify Goals: Determine the primary goals of your analysis, such as understanding customer behavior, evaluating a product’s effectiveness, or assessing market trends.
- Formulate Questions: Develop specific questions that will guide your analysis and help you focus on relevant data.
Clear objectives provide direction for the analysis and ensure that the results align with your needs.
2. Choosing the Right Data
Selecting the right data is crucial for effective data analysis. Ensure that the data you choose is relevant, accurate, and sufficient for your objectives.
- Data Sources: Identify reliable sources of data, such as surveys, databases, or observational studies.
- Data Quality: Assess the quality of the data, including its accuracy, completeness, and relevance.
High-quality data is the cornerstone of reliable data analysis and contributes to meaningful insights.
3. Data Cleaning and Preparation
Data cleaning and preparation involve organizing and refining the data to make it suitable for analysis. This step addresses common issues such as missing values, inconsistencies, and errors.
- Handle Missing Values: Decide how to address missing values, either by imputing them with estimated values or removing affected records.
- Remove Duplicates: Identify and eliminate duplicate records to avoid skewed results.
- Correct Errors: Check for and correct errors in the data, such as typos or incorrect entries.
Proper data cleaning ensures that the analysis is based on accurate and reliable information.
4. Exploratory Data Analysis (EDA)
Exploratory Data Analysis (EDA) is an initial step in data analysis that involves examining the data to uncover patterns, trends, and relationships.
- Descriptive Statistics: Calculate basic statistics, such as mean, median, mode, and standard deviation, to summarize the data.
- Data Visualization: Use graphs and charts, such as histograms, scatter plots, and box plots, to visualize data distributions and relationships.
EDA provides a comprehensive understanding of the data and guides further analysis.
5. Applying Statistical Methods
Applying statistical methods involves using mathematical techniques to analyze the data and draw conclusions. Choose appropriate methods based on the type of data and research questions.
- Inferential Statistics: Use techniques such as hypothesis testing, confidence intervals, and regression analysis to make inferences about the population based on the sample data.
- Predictive Analytics: Employ methods like linear regression or machine learning algorithms to predict future outcomes based on historical data.
Statistical methods provide the tools to analyze data rigorously and derive meaningful insights.
6. Interpreting Results
Interpreting the results involves translating the findings from the analysis into actionable insights and recommendations.
- Contextualize Findings: Relate the results to the research questions and objectives, considering the implications for decision-making.
- Communicate Insights: Present the findings in a clear and understandable manner, using visualizations and summaries to convey key points.
Effective interpretation ensures that the results are meaningful and relevant to stakeholders.
Strategies for Mastering Data Analysis
Mastering data analysis involves implementing practical strategies to enhance your analytical skills and achieve better results. Here are some key strategies to consider:
1. Develop a Structured Approach
Adopt a structured approach to data analysis by following a systematic process. This includes defining objectives, preparing data, conducting exploratory analysis, applying statistical methods, and interpreting results.
- Create a Plan: Develop a detailed plan outlining each step of the analysis process.
- Follow Best Practices: Adhere to best practices for data collection, cleaning, and analysis to ensure accuracy and reliability.
A structured approach helps in maintaining consistency and ensures that all aspects of the analysis are covered.
2. Leverage Advanced Tools and Software
Utilize advanced tools and software to streamline data analysis and handle complex datasets efficiently.
- Statistical Software: Use tools such as SPSS, R, or Python for performing sophisticated statistical analyses and managing large datasets.
- Data Visualization Tools: Employ tools like Tableau or Power BI to create interactive and informative visualizations.
Advanced tools and software enhance your ability to analyze data effectively and gain deeper insights.
3. Focus on Data Visualization
Data visualization is a powerful way to communicate findings and make complex data more accessible. Effective visualizations can reveal patterns and trends that might be missed in raw data.
- Choose Appropriate Visualizations: Select visualizations that best represent the data and highlight key findings. For example, use scatter plots for relationships between variables and bar charts for categorical comparisons.
- Design for Clarity: Ensure that visualizations are clear, with appropriate labels, scales, and legends.
Well-designed visualizations improve the clarity and impact of your data analysis.
4. Validate Your Findings
Validate your findings to ensure that the results are accurate and reliable. This involves checking for consistency and verifying that the analysis is free from errors or biases.
- Cross-Check Results: Compare findings with other studies or datasets to verify consistency.
- Perform Sensitivity Analysis: Test how changes in data or methods affect the results to assess the robustness of your findings.
Validation enhances the credibility of your data analysis and supports more reliable conclusions.
5. Keep Learning and Adapting
Stay updated with the latest developments in data analysis methodologies and tools. Continuous learning and adaptation are essential for improving your analytical skills and keeping pace with advancements in the field.
- Attend Workshops and Conferences: Participate in events to learn about new techniques and best practices.
- Explore Online Resources: Utilize online courses, tutorials, and forums to expand your knowledge and skills.
Ongoing learning helps you stay proficient in data analysis and adapt to evolving trends and technologies.
Real-World Applications of Data Analysis
Data analysis has a wide range of applications across various industries, providing valuable insights and supporting decision-making.
1. Healthcare
In healthcare, data analysis is used to improve patient care, optimize treatments, and analyze health trends.
- Patient Outcomes: Analyze data from clinical trials and patient records to evaluate treatment effectiveness and identify factors influencing health outcomes.
- Predictive Modeling: Use predictive analytics to forecast disease outbreaks or patient admissions.
Data analysis supports evidence-based practices and enhances healthcare delivery.
2. Marketing
In marketing, data analysis helps businesses understand consumer behavior, evaluate campaign performance, and optimize strategies.
- Customer Segmentation: Analyze customer data to identify segments and tailor marketing efforts to specific groups.
- Campaign Evaluation: Assess the effectiveness of marketing campaigns by analyzing metrics such as conversion rates and ROI.
Data analysis drives targeted marketing strategies and improves campaign outcomes.
3. Finance
In finance, data analysis is crucial for managing risk, optimizing investments, and analyzing financial performance.
- Risk Management: Analyze financial data to assess and manage risks, including credit risk and market risk.
- Investment Analysis: Use statistical methods to evaluate investment opportunities and forecast market trends.
analysis supports informed financial decision-making and enhances investment strategies.
data analysis
Mastering data analysis is key to unlocking valuable insights and making informed decisions. By understanding the core components of data , implementing practical strategies, and leveraging advanced tools, you can simplify the complexities of working with numbers and achieve better results. Embrace the power of analysis to transform raw data into actionable insights and drive success in your research, business, or any other field. Whether you’re exploring healthcare trends, optimizing marketing strategies, or analyzing financial performance, effective data provides the foundation for informed decision-making and meaningful outcomes.